Our Lunch Seminar series continues this week! We are very looking forward to having Riccardo Guidotti (University of Pisa) next Wednesday (October 27), starting from 1 PM. His talk on Auto-Encoders for Explainable AI matches very well with the Machine Ethics seminar given by Marija two weeks ago. Make sure you don’t miss this one out if you want to know more about these exciting topics in Artificial Intelligence. See below for the link and more information.
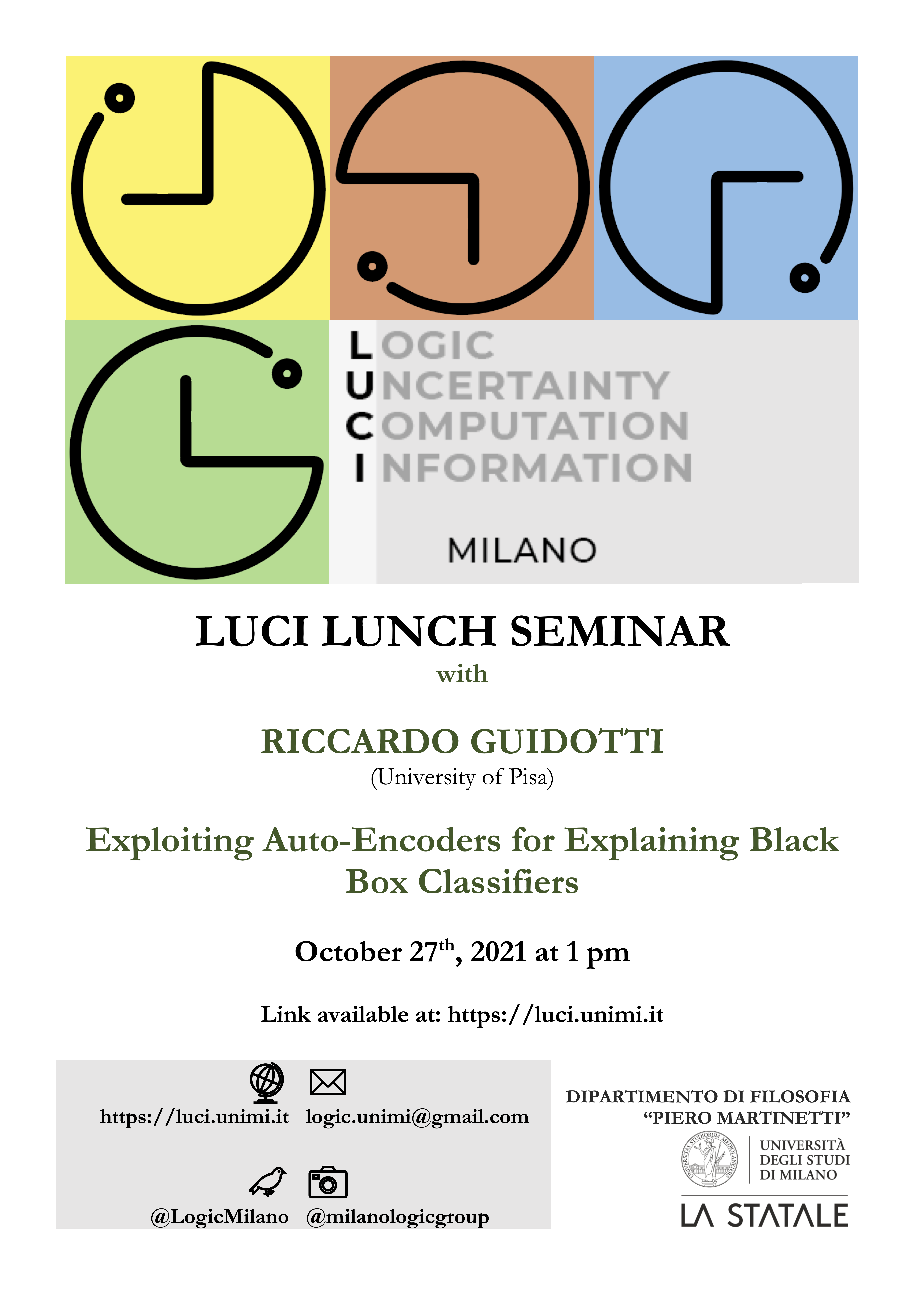
Title: Exploiting Auto-Encoders for Explaining Black Box Classifiers
Abstract: Artificial Intelligence (AI) is nowadays one of the most important scientific and technological areas, with a tremendous socio-economic impact and a pervasive adoption in every field of the modern society. Many applications in different fields, such as credit score assessment, medical diagnosis, autonomous vehicles, and spam filtering are based on AI decision systems. Unfortunately, these systems often reach their impressive performance through obscure machine learning models that “hide” the logic of their internal decision processes to humans because not humanly understandable. For this reason, these models are called black box models, i.e., models used by AI to accomplish a task for which either the logic of the decision process is not accessible, or it is accessible but not human-understandable. Examples of machine learning black box models adopted by AI systems include Deep Neural Networks, Ensemble classifiers, and so on. The missing interpretability of black box models is a crucial issue for ethics and a limitation to AI adoption in socially sensitive and safety-critical contexts such as healthcare and law. As a consequence, the research in eXplainable AI (XAI) has recently caught much attention and there has been an ever-growing interest in this research area to provide explanations on the behavior of black box models. A promising line of research in XAI exploits auto-encoders for explaining black box classifiers working on non-tabular data (e.g., images, time series, and texts). The ability of autoencoders to compress any data in a low-dimensional tabular representation, and then reconstruct it with negligible loss, provides the great opportunity to work in the latent space for the extraction of meaningful explanations, for example through the generation of new synthetic samples, consistent with the input data, that can be fed to a black-box to understand where its decision boundary lies. In this presentation we discuss recent XAI solutions based on autoencoders that enable the extraction of meaningful explanations composed by factual and counterfactual rules, and by exemplar and counter-exemplar samples, offering a deep understanding of the local decision of the black box.
Short Bio: Riccardo Guidotti is currently an Assistant Professor (RTD-B) at the Department of Computer Science University of Pisa, Italy, and a member of the Knowledge Discovery and Data Mining Laboratory (KDDLab), a joint research group with the Information Science and Technology Institute of the National Research Council in Pisa. In 2013 and 2010 he graduated cum laude in Computer Science (MS and BS) at the University of Pisa. He received a PhD in Computer Science with a thesis on Personal Data Analytics in the same institution. He won the IBM fellowship program and has been an intern in IBM Research Dublin, Ireland in 2015. His research interests are in personal data mining, clustering, explainable models, analysis of transactional data.
Zoom link: https://us02web.zoom.us/j/86952228423