Algorithmic Bias
In a handful of years, AI has made its presence known, beyond the scientific and academic fields, in everyday life of ordinary people, and the risks associated with this technology have become evident while solutions are being sought.
In particular, Algorithmic Bias refers to the phenomenon whereby an algorithmic system produces distorted, partial or unfair results towards some groups of people (for example, women, ethnic minorities or people of a certain age group) or individuals.
As algorithms increasingly impact key areas such as healthcare, criminal justice, creditworthiness, and access to education, understanding and addressing algorithmic bias is essential for the development of more equitable and inclusive systems.
BRIO
Bias detection software based on TPTND (Trustworthy Probabilistic Typed Natural Deduction) logic
Data Fairness Analysis: comparing the behaviour of the AI system against a desirable one
Model Fairness Analysis: comparing the behaviour of the AI system with respect to a sensitive group against another sensitive group related to the same feature
Risk Analysis: a measure of the number of datapoints where a test of type FreqVsFreq or FreqVsRef fails, of how far it is from not failing on some datapoints, and of how difficult was it for it to fail
Bias Amplifications Chains and Loops
ML systems not only reproduce biases and stereotypes but, even more worryingly, amplify and reinforce them. Bias amplification occurs across the entire ML pipeline, creating bias amplification chains (from society to model output, through to the training set) and loops (mitigation operations are usually performed by other ML systems)
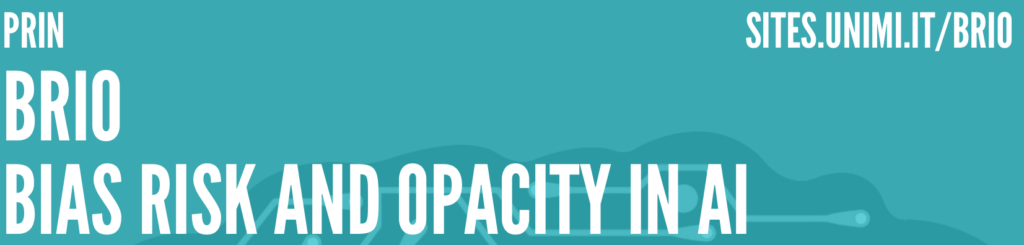